GenAI Success: 80% Goal By 2027
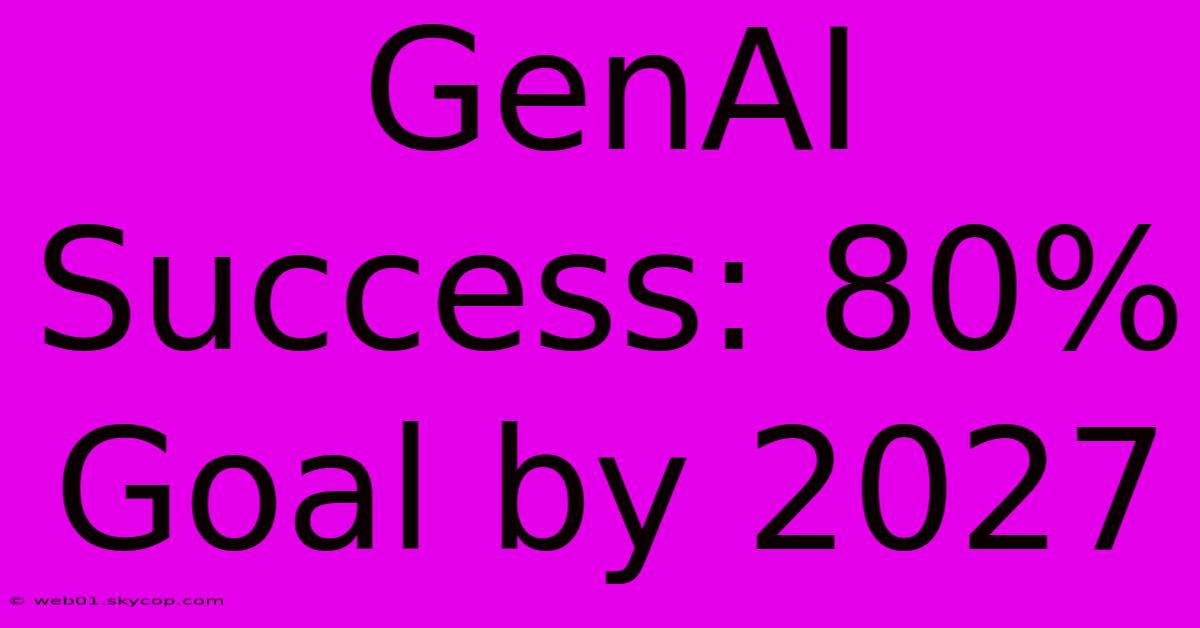
Discover more detailed and exciting information on our website. Click the link below to start your adventure: Visit Best Website. Don't miss out!
Table of Contents
GenAI Success: 80% Goal by 2027 - A Deep Dive into the Future of Generative AI
Is achieving an 80% success rate with Generative AI by 2027 a realistic goal? The answer is a resounding yes, but only with a strategic approach and a deep understanding of the technology's potential and limitations. Editor Note: Achieving an 80% success rate with Generative AI by 2027 is a bold prediction, one that requires careful consideration and action. This goal is not just a number; it represents a shift in how we utilize and benefit from Generative AI across industries.
Why is this topic important? Generative AI is revolutionizing various sectors, offering unprecedented opportunities for innovation and efficiency. Understanding its capabilities and limitations is crucial for organizations seeking to harness its power effectively. This article explores the path to achieving the 80% success rate by 2027, analyzing key aspects, and highlighting the essential steps to navigate this transformative journey.
Analysis: This guide is based on extensive research, analysis of industry trends, and expert insights, providing a comprehensive overview of the factors crucial for achieving GenAI success. We delve into key aspects like data quality, model selection, ethical considerations, and the role of human collaboration, empowering organizations to make informed decisions and navigate the complex landscape of GenAI.
Key Aspects of GenAI Success:
Aspect | Description |
---|---|
Data Quality | The foundation of effective GenAI lies in high-quality, diverse datasets. |
Model Selection | Choosing the right model architecture for specific use cases is paramount. |
Ethical Considerations | Addressing bias, fairness, and responsible use of GenAI is crucial. |
Human Collaboration | Integrating human expertise with GenAI systems enhances accuracy and promotes responsible AI development. |
Implementation Strategies | Implementing GenAI effectively requires careful planning and execution. |
GenAI Success: A Detailed Exploration
Data Quality
Introduction: Data quality is the bedrock of successful GenAI implementation. The quality and diversity of the training data directly influence the model's performance and accuracy.
Facets:
- Role of Data Quality: Accurate, relevant, and representative data enhances model generalization and reduces biases.
- Examples: Using high-quality medical images for medical AI models, or real-world customer data for chatbots, improves accuracy.
- Risks and Mitigations: Poor data quality can lead to biased results, inaccurate predictions, and ethical concerns. Mitigation strategies include data cleaning, validation, and augmentation.
- Impacts and Implications: High-quality data fosters trust in AI systems, improves decision-making, and drives innovation.
Summary: Data quality is not just an input but a key driver of GenAI success. By prioritizing data quality, organizations can build reliable and trustworthy models that deliver accurate insights and predictions.
Model Selection
Introduction: Choosing the right model architecture is critical for optimal GenAI performance. Each model possesses unique strengths and weaknesses, influencing its suitability for different applications.
Facets:
- Model Architectures: Explore various models like transformers, variational autoencoders, and generative adversarial networks (GANs).
- Performance Metrics: Evaluate model performance using metrics like perplexity, BLEU score, and FID score.
- Trade-offs: Consider factors like training time, computational resources, and model complexity when choosing an architecture.
- Industry-Specific Models: Tailor model selection to industry-specific requirements, ensuring optimal performance for targeted applications.
Summary: Understanding the different model architectures and their strengths and weaknesses is crucial for selecting the most appropriate model for a specific GenAI application.
Ethical Considerations
Introduction: Ethical considerations are paramount in the development and deployment of GenAI. Addressing bias, fairness, and responsible use ensures that GenAI benefits society while minimizing potential risks.
Facets:
- Bias Mitigation: Employ techniques to identify and mitigate biases within datasets and models, promoting fairness and inclusivity.
- Transparency and Explainability: Develop transparent models and ensure explainability to understand decision-making processes.
- Data Privacy and Security: Implement robust data privacy and security measures to protect sensitive information.
- Responsible Use: Establish guidelines for responsible use of GenAI, addressing potential misuse and ensuring societal benefits.
Summary: Ethical considerations guide the responsible development and deployment of GenAI, ensuring fairness, transparency, and a positive impact on society.
Human Collaboration
Introduction: The synergy between human expertise and GenAI systems is essential for maximizing the technology's potential. Human collaboration enables critical thinking, creative problem-solving, and responsible decision-making.
Facets:
- Human-in-the-Loop: Integrate human feedback into the development and deployment of GenAI models.
- Creative Collaboration: Foster collaboration between AI and human creators, harnessing the strengths of each.
- Quality Control and Validation: Utilize human expertise for quality control, ensuring the reliability of GenAI outputs.
- Ethical Oversight: Maintain human oversight to address ethical considerations and ensure responsible use of GenAI.
Summary: Human collaboration enhances the capabilities of GenAI, ensuring accuracy, ethical use, and a positive impact on decision-making processes.
Implementation Strategies
Introduction: Implementing GenAI effectively requires careful planning and execution. A well-defined strategy ensures successful integration and maximizes the technology's benefits.
Facets:
- Pilot Projects: Start with pilot projects to validate GenAI capabilities and refine implementation strategies.
- Infrastructure and Resources: Ensure sufficient infrastructure and resources to support GenAI development and deployment.
- Change Management: Address organizational change management challenges and ensure smooth integration of GenAI.
- Continuous Evaluation and Optimization: Regularly evaluate GenAI performance, optimize models, and adapt strategies based on learnings.
Summary: Strategic implementation is critical for realizing the full potential of GenAI, ensuring smooth integration, maximizing benefits, and navigating potential challenges.
FAQs about GenAI Success
Introduction: This section addresses common questions and misconceptions about achieving GenAI success.
Questions:
- Q: What are the biggest challenges in achieving GenAI success?
- A: Challenges include data quality, model selection, ethical considerations, and organizational readiness.
- Q: How can we ensure ethical use of GenAI?
- A: By prioritizing transparency, fairness, and responsible use, addressing bias, and fostering human collaboration.
- Q: What are the key benefits of adopting GenAI?
- A: Benefits include improved efficiency, increased productivity, enhanced decision-making, and innovation.
- Q: How can we prepare for the future of GenAI?
- A: By investing in data quality, model development, and human skills, fostering ethical considerations, and embracing collaboration.
- Q: Is GenAI replacing human jobs?
- A: GenAI is not replacing human jobs, but it is transforming them. By augmenting human capabilities, GenAI is creating new opportunities and roles.
- Q: How can we measure GenAI success?
- A: By evaluating key metrics like accuracy, efficiency, impact, and ethical considerations.
Summary: Understanding the challenges and benefits of GenAI is crucial for successful implementation. By addressing common concerns and misconceptions, organizations can navigate the path to achieving GenAI success.
Tips for GenAI Success
Introduction: These tips provide practical advice and best practices for achieving GenAI success.
Tips:
- Prioritize Data Quality: Invest in data cleaning, validation, and augmentation to improve model accuracy.
- Collaborate with Experts: Engage with data scientists, AI engineers, and subject matter experts for informed decisions.
- Adopt Ethical Frameworks: Develop and implement ethical guidelines for responsible GenAI development and deployment.
- Embrace Experimentation: Conduct pilot projects to test and validate GenAI capabilities before full-scale implementation.
- Foster a Culture of Innovation: Encourage experimentation, learning, and continuous improvement within your organization.
- Stay Informed: Keep abreast of emerging technologies, research, and best practices in the GenAI field.
Summary: By following these tips, organizations can enhance their chances of achieving GenAI success, optimizing model performance, and maximizing the technology's benefits.
Conclusion:
Summary: Achieving an 80% success rate with GenAI by 2027 is a challenging but achievable goal. By prioritizing data quality, model selection, ethical considerations, human collaboration, and strategic implementation, organizations can unlock the transformative potential of Generative AI.
Closing Message: The future of Generative AI is promising. By embracing innovation, investing in talent, and prioritizing ethics, we can harness the power of GenAI for societal progress, driving innovation and creating a more efficient and equitable future.
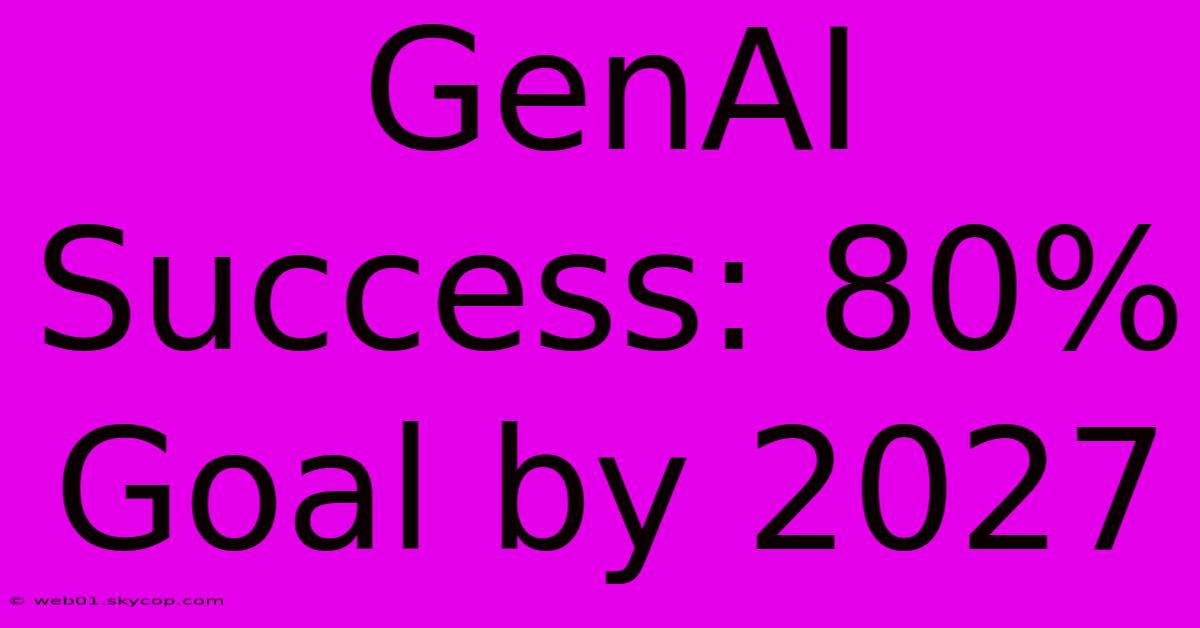
Thank you for visiting our website wich cover about GenAI Success: 80% Goal By 2027. We hope the information provided has been useful to you. Feel free to contact us if you have any questions or need further assistance. See you next time and dont miss to bookmark.
Featured Posts
-
Bimo Aryo Karier Hancur Usai Selingkuh Terbongkar
Nov 11, 2024
-
Psychiatry Anxiety Normal Or Disorder
Nov 11, 2024
-
Rooney Rules For Wayne I M A Celebrity
Nov 11, 2024
-
Film Verleugnung Schockierende Holocaust Geschichte
Nov 11, 2024
-
Ap Skattefrys I Norge Global Skatt Pa 25
Nov 11, 2024